Unlocking the Future of Finance: How Retrieval-Augmented Generation (RAG) is Revolutionizing Financial Services
In today’s fast-paced financial services industry, the need for advanced technologies to manage vast amounts of data and make informed decisions is greater than ever. One such emerging technology is Retrieval-Augmented Generation (RAG), a powerful AI framework that combines the strengths of retrieval-based systems and generative models. It’s like having an all-star team of data analysts and advisors rolled into one. Let’s dive into what RAG is, how it’s being applied in finance, and why it’s becoming indispensable.
How Does RAG Work?
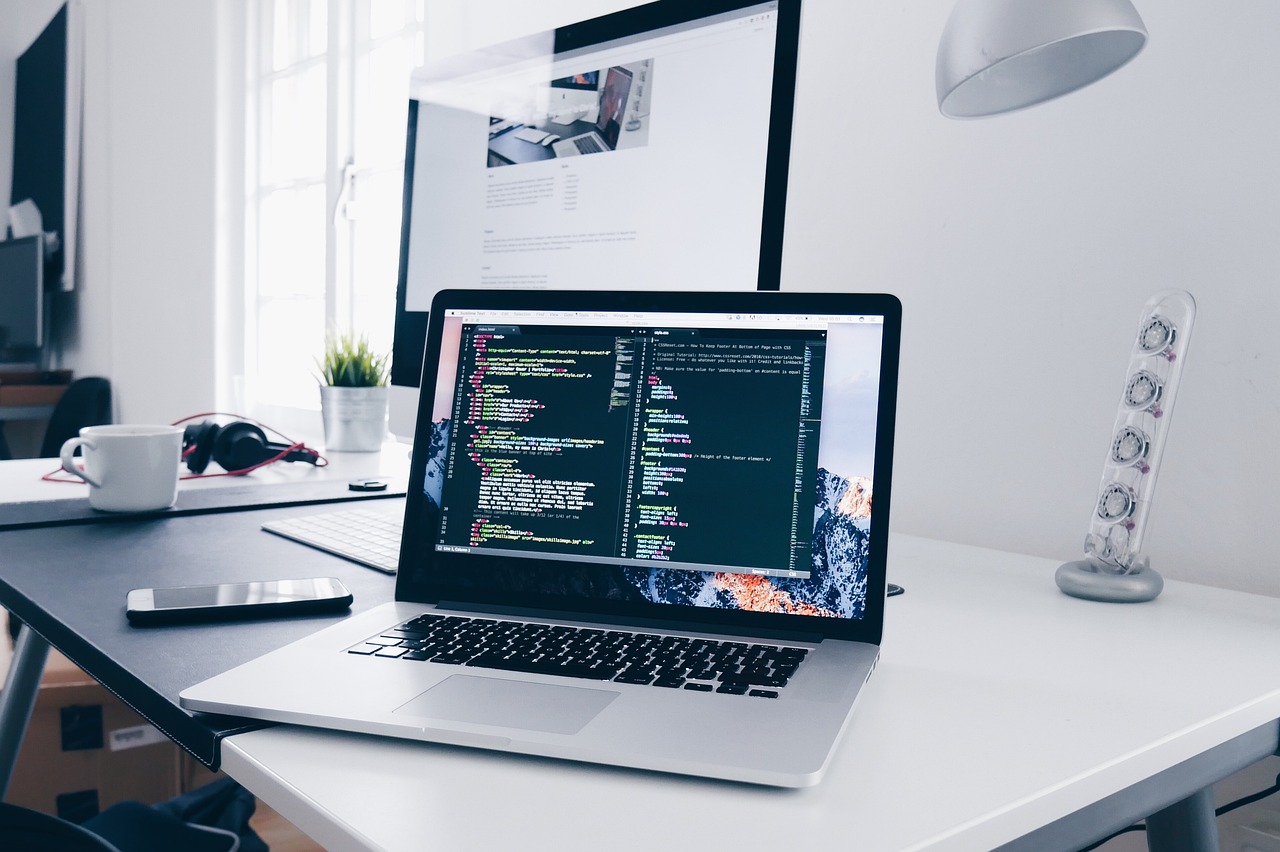
RAG is a framework in artificial intelligence designed to enhance the quality of responses produced by large language models (LLMs) by integrating external sources of knowledge. This approach supplements the internal information of the LLM with external data.
If your LLM is like an eager chef who’s mastered basic cooking techniques but needs a little help perfecting their sushi skills, RAG is like giving them a detailed sushi recipe straight from a top Japanese chef.
RAG, is a way to provide AI models with a solid external reference to improve the quality of their responses. Here’s how it works:
Retrieval: Say you have a few key recipes from a renowned sushi master. With RAG, you direct the model to pull information from these specific recipes.
Augmented: You then tweak the model’s prompt to ensure it consults these expert recipes. RAG acts as a form of prompt engineering—in this case, you’re guiding the AI to learn the nuances of sushi-making.
Generation: With the right information at its fingertips, the model can now whip up a response that’s accurate and well-informed, just like a chef creating sushi that’s authentic and expertly crafted.
RAG is also a practical and cost-effective approach compared to other methods like fine-tuning. Instead of retraining the entire model, you can combine an existing LLM with external expert resources to achieve better outcomes—like handing the chef the sushi recipe rather than re-teaching them how to cook from scratch.
Transformative Impact in Financial Services: Key Use Cases
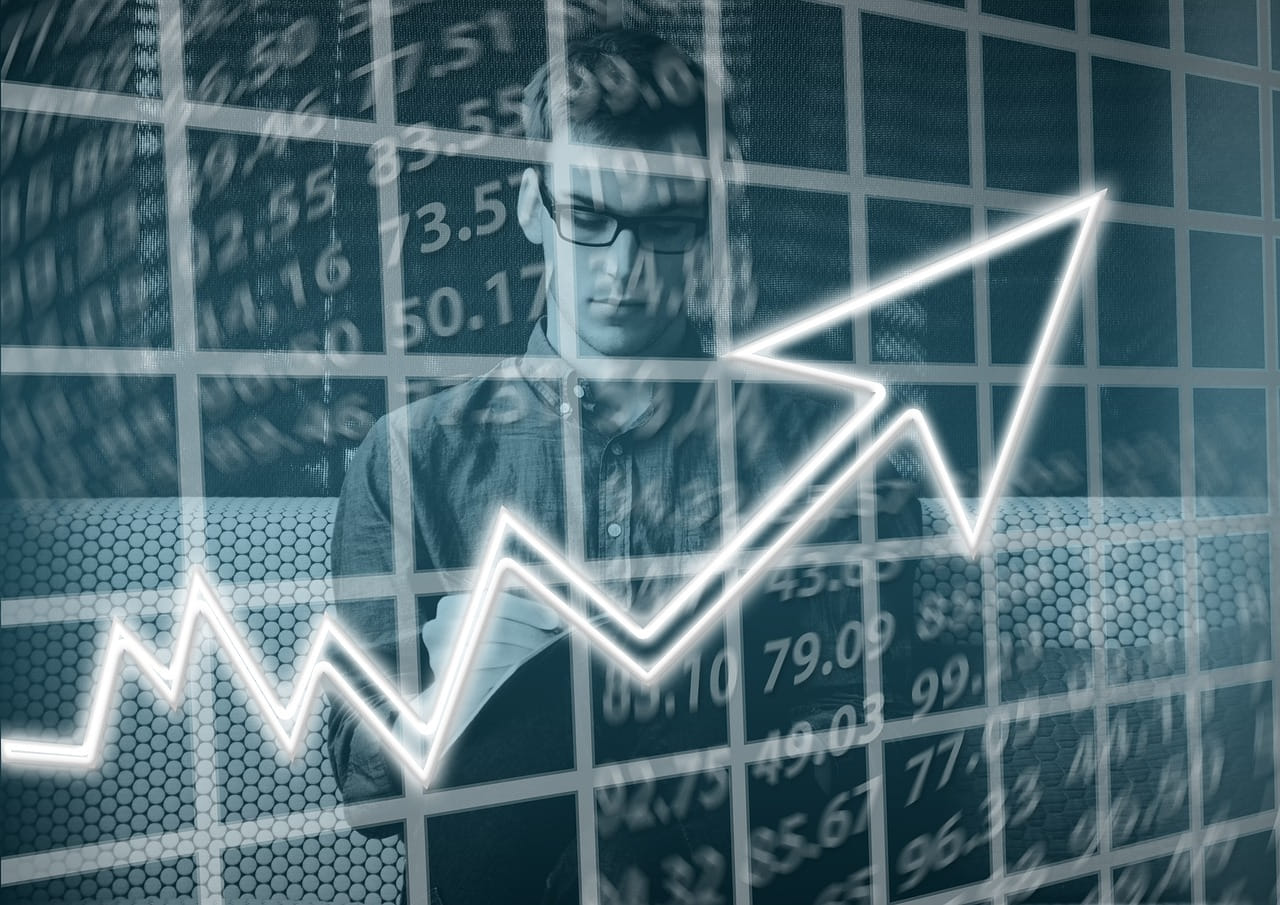
The financial services industry , with its reliance on data-driven decision-making, is an ideal candidate for RAG applications. Below are three potential use cases where RAG can significantly impact the sector:
Risk Management and Compliance: In the highly regulated financial industry, staying compliant with evolving regulations is crucial. RAG can be used to enhance risk management by retrieving relevant regulatory documents, historical data, and case studies to assist in decision-making. For example, when evaluating the risk of a new financial product, RAG can retrieve similar cases and regulations that apply, helping compliance officers make informed decisions and avoid potential legal issues.
Customer Support and Virtual Assistants: Financial institutions often use chatbots and virtual assistants to handle customer inquiries. However, these systems can struggle with complex or nuanced questions. By integrating RAG, these virtual assistants can retrieve relevant documents, policy details, and historical customer interactions to provide more accurate and contextually appropriate responses. This improves customer satisfaction by resolving queries more effectively and reducing the need for human intervention.
Investment Research and Analysis: Investment professionals need access to vast amounts of information to make informed decisions. RAG can be utilized to automate the process of retrieving relevant financial reports, news articles, and market analyses, which can then be used to generate detailed investment recommendations. By leveraging RAG, financial analysts can quickly access the most relevant data, enabling them to make better-informed decisions in a shorter amount of time.
Key Benefits of Integrating RAG in Financial Services
The integration of RAG into financial services offers several key benefits:
Enhanced Decision-Making: By combining the precision of retrieval-based methods with the creativity of generative models, RAG enables more accurate and contextually relevant decision-making. This is particularly valuable in complex fields like risk management and investment analysis, where access to the right information is critical.
Improved Customer Experience: RAG-powered virtual assistants and chatbots can provide more accurate and personalized responses, leading to higher customer satisfaction. By effectively addressing customer needs, financial institutions can build stronger relationships with their clients.
Operational Efficiency: Automating information retrieval and generation processes can significantly reduce the time and resources required for tasks such as compliance checks, investment research, and customer support. This leads to increased operational efficiency and cost savings for financial institutions.
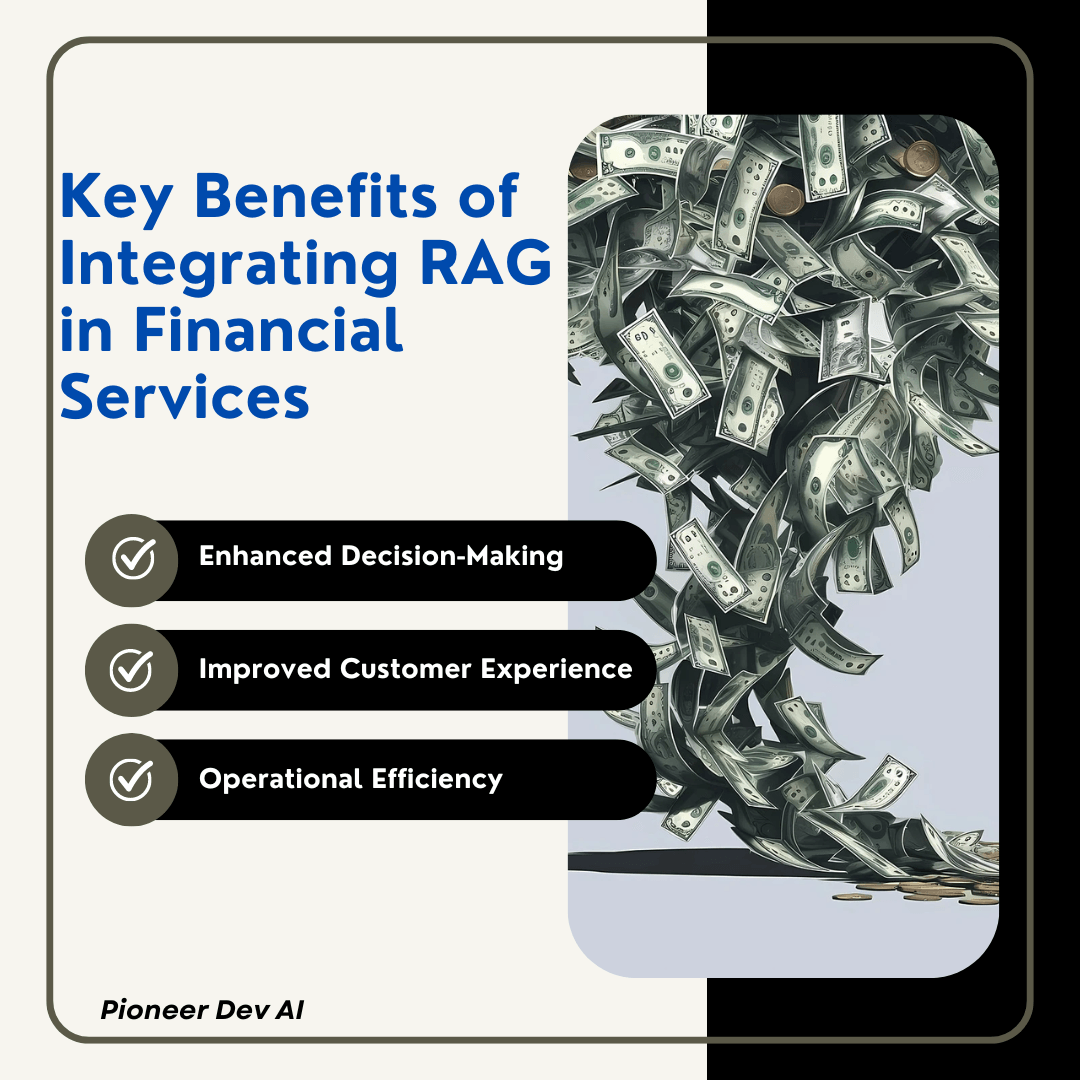
Conclusion
As the financial services industry continues to evolve, the adoption of advanced AI technologies like RAG will play a pivotal role in enhancing decision-making, improving customer experiences, and optimizing operations. Pioneer Dev AI is at the forefront of this transformation, offering specialized AI solutions tailored to the unique needs of the finance industry. From building RAG-powered applications to developing chatbots, generative AI models, and full-stack SaaS platforms, Pioneer Dev AI delivers high-performance, user-centric solutions that enable financial institutions to stay ahead of the curve. By integrating AI into web applications, Pioneer Dev AI helps clients unlock the full potential of AI, making their products more efficient, user-friendly, and impactful in the competitive financial landscape.